TL;DR
We present an text-guided 3DGS scene editing pipeline with multi-view and illumination consistency during generation.
Abstract
Recent text-guided generation of individual 3D objects has achieved great success using diffusion priors. However, when directly applied to scene editing tasks (e.g., object replacement, object insertion), these methods lack the context of global information, leading to inconsistencies in illumination or unrealistic occlusion.
To bridge the gap, we introduce an illumination-aware 3D scene editing pipeline for 3D Gaussian Splatting (3DGS) representation.
Our key observation is that inpainting by the state-of-the-art conditional 2D diffusion model excels at filling missing regions while considering the context. In this paper, we introduce the success of 2D diffusion inpainting to 3D scene object replacement. Specifically, our approach automatically picks the representative views that best express the global illumination, and these views are inpainted using the state-of-the-art 2D diffusion model. We further developed a coarse-to-fine optimization pipeline that takes the inpainted representative views as input and converts them into 3D Gaussian splatting to achieve scene editing. To acquire an ideal inpainted image,
we introduce an Anchor View Proposal (AVP) algorithm to find a single view that best represents the scene illumination in target region. In the coarse-to-fine 3D lifting component, we first achieve image-to-3D lifting given an ideal inpainted view.
In the fine step of Texture Enhancement, we introduce a novel Depth-guided Inpainting Score Distillation Sampling (DI-SDS), which enhances geometry and texture details with the inpainting diffusion prior, beyond the scope of the 3D-aware diffusion prior knowledge in the first coarse step.
DI-SDS not only provides fine-grained texture enhancement, but also urges optimization to respect scene lighting.
Our approach efficiently achieves local editing with global illumination consistency and we demonstrate robustness of our method by evaluating editing in real scenes containing explicit highlight and shadows, and compare against the state-of-the-art text-to-3D editing methods.
Method Overview
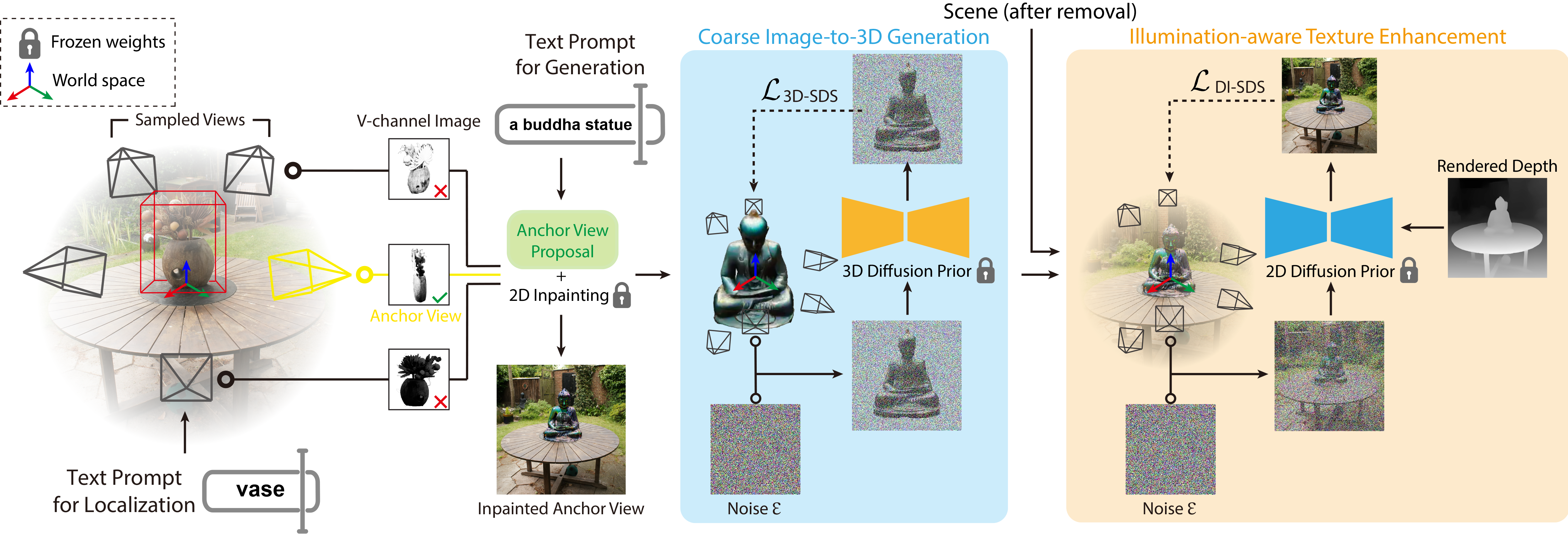
Citation
@misc{xiao2024localizedgaussiansplattingediting,
title={Localized Gaussian Splatting Editing with Contextual Awareness},
author={Hanyuan Xiao and Yingshu Chen and Huajian Huang and Haolin Xiong and Jing Yang and Pratusha Prasad and Yajie Zhao},
year={2024},
eprint={2508.00083},
archivePrefix={arXiv},
primaryClass={cs.CV},
url={https://arxiv.org/abs/2508.00083},
}